10B Agentic Finance Vertical SaaS
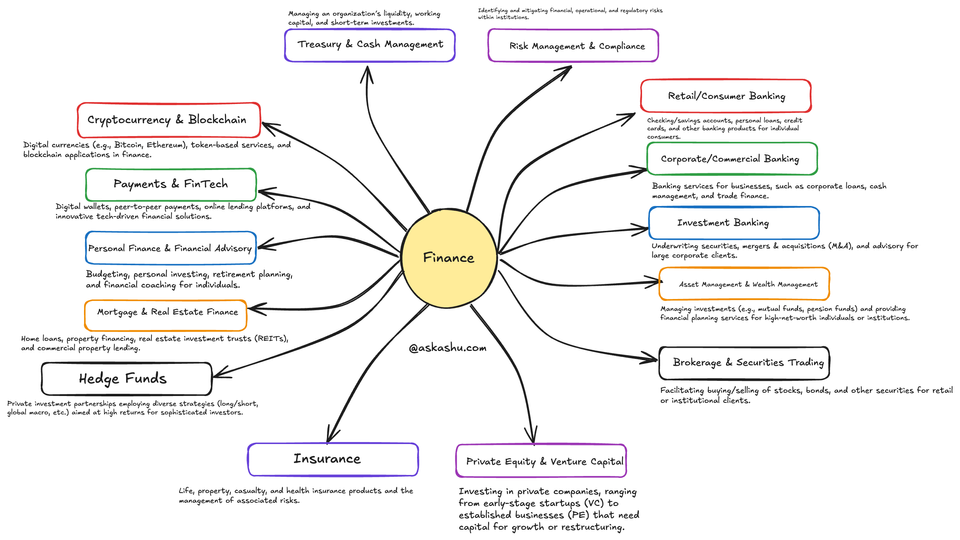
one billion one person company will happen - Sam Altman
The finance industry is complex, fragmented, and deeply reliant on expertise and I believe this vertical has 10B opportunity, if some one dares enough.
To build agentic Vertical SaaS for finance, we first need to define the problem space—what challenges do AI agents need to solve?
The mind map, in the title, is an attempt to break down the 14 major financial verticals, from investment banking and hedge funds to payments, compliance, and wealth management. Each of these areas presents unique pain points, requiring domain-specific intelligence and automation. We are still in the early days of agentic AI and Vertical SaaS. But if we get it right, the potential impact is massive.
To take stepped approach , in we can categorise these verticals in the diagram in three categories - Low-Hanging, Medium, and Hard Nut—based on the relative ease or complexity of implementing AI agents. I considered factors such as data availability, regulatory constraints, domain complexity, and the maturity of existing AI solutions.
Low-Hanging Fruit
These verticals tend to have high data availability, well-defined processes, and relatively lower regulatory hurdles compared to other areas. AI agents can often be deployed quickly for immediate gains in automation and customer experience.
- Retail/Consumer Banking High volume of standardised transactions, large customer datasets, and well-established processes (e.g., account management, credit card applications).
AI Application: Chatbots for customer service, predictive analytics for personalised product recommendations, and basic fraud detection.
- Personal Finance & Financial Advisory Less complex product offerings (e.g., budgeting, savings tools) and fewer regulatory complications than institutional finance.
AI Application: Robo-advisors for portfolio suggestions, expense tracking, and financial planning chatbots.
- Payments & FinTech Mature ecosystem of payment gateways and digital wallets with relatively standardised APIs and protocols.
AI Application: Real-time fraud detection, payment automation, transaction analytics.
- Treasury & Cash Management Standardised cash flow operations and forecasting tasks that benefit from straightforward predictive modeling.
AI Application: Liquidity forecasting, automated treasury operations, and basic anomaly detection in cash flow patterns.
Medium Complexity
These verticals have moderate regulatory requirements or specialised processes, requiring a deeper understanding of financial rules, but still benefit from relatively well-structured data and existing frameworks for AI.
- Corporate/Commercial Banking Larger-scale transactions and credit assessments than retail banking, but with more standardised underwriting processes than investment banking.
AI Application: Automated loan underwriting, risk scoring for corporate clients, cash flow forecasting for businesses.
- Insurance Complex underwriting and claims processes, but insurance has a long history of using statistical models (actuarial science), making AI adoption smoother.
AI Application: Fraud detection, automated claims processing, personalised policy recommendations.
- Mortgage & Real Estate Finance Heavy documentation and regulatory checks, but much of the process (e.g., appraisals, credit checks) is well-defined and data-driven.
AI Application: Automated property valuation, streamlined mortgage approvals, document scanning with NLP.
Asset Management & Wealth Management Involves advanced analytics for portfolio optimisation but typically deals with more transparent asset classes than hedge funds or private equity.
AI Application: Robo-advisors for portfolio rebalancing, performance attribution analysis, personalised investment strategies.
- Risk Management & Compliance Heavily regulated, but also well-established frameworks (e.g., Basel accords) and data sources that support analytics.
AI Application: Real-time compliance monitoring, anomaly detection in transactions, automated reporting and audit trails.
Hard Nut to Crack
These verticals involve highly specialised or complex financial products, intensive regulatory scrutiny, or less-structured data environments, making the development and deployment of AI agents significantly more challenging.
- Investment Banking Complex deal structures (M&A, IPOs), sophisticated financial modeling, and sensitive, often confidential data.
AI Application: Deal sourcing and due diligence analytics, predictive modeling for capital markets, sentiment analysis on market-moving news.
- Hedge Funds Proprietary trading strategies, highly complex and often high-frequency data, and intense pressure for alpha generation.
AI Application: Algorithmic trading using reinforcement learning, predictive market analytics, and alternative data signal extraction.
- Brokerage & Securities Trading Real-time trading execution with significant regulatory oversight (e.g., SEC, MiFID II), requiring ultra-low latency and robust risk controls.
AI Application: Automated order execution, dynamic hedging strategies, real-time risk monitoring.
- Private Equity & Venture Capital Involves analyzing private, less-structured data (pitch decks, company financials, market projections), plus complex deal structuring.
AI Application: Automated screening of investment targets, valuation modeling, predictive analytics for exit strategies.
- Cryptocurrency & Blockchain Rapidly evolving technology, volatile markets, and uncertain regulatory landscapes across different jurisdictions.
AI Application: Smart contract audits, real-time market analytics for highly volatile assets, anomaly detection for illicit transactions.
The roadmap to a $10B agentic Vertical SaaS for finance is not just about automation—it's about rethinking how financial intelligence is codified, scaled, and executed.
By systematically tackling low-hanging opportunities while gradually advancing into high-complexity domains, we can build AI-driven financial agents that are not just tools, but indispensable collaborators in the industry. The ultimate goal? To create AI agents that think, reason, and execute at an expert level, reducing inefficiencies, democratising financial services, and unlocking unprecedented value.
We are still in the early innings of this transformation. But if history has taught us anything, it’s that industry-defining companies are built by those willing to challenge the status quo.
The one-billion-dollar, one-person company might be an inevitability—but a $10B AI-driven financial Vertical SaaS is an opportunity waiting to be seized. Who’s ready to build? Feel free to DM.
Member discussion